Gain a competitive advantage with advanced AML solutions powered by AI and ML
2021 was a blockbuster year for the regulators. An estimated $ 2.7 billion was raised in fines or anti-money laundering (AML) and Know Your Customer (KYC) violations in the first half of 2021 itself. The number of institutions that were fined quadrupled from 24 in 2020 to 80 in 2021.
There was a diverse list of defaulters last year, something not seen earlier.
- There was a bank holding company specializing in credit cards, auto loans, banking, and saving accounts.
- A fintech that achieved phenomenal growth for trading
- A cryptocurrency platform
All failed to meet AML compliance standards and were fined. - Credit Suisse, the Global Investment Bank, was another major defaulter.
All this is an indication that the regulators’ tolerance for default is very limited.
And secondly, the variance in defaulter listing also indicates once and for all that the ambit for AML breaches has widened. No longer confined to the big banks only, post covid-19 all financial institutions must comply with the new reforms enforced by the Financial Crimes Enforcement Network (FinCEN), Financial Action Task Force (FATF), and Office of Foreign Assets Control (OFAC) or face the heat.
What are the key challenges that banks and fintechs face regarding AML compliance?
Considering that AML observance is mandatory why do banks and financial institutions fail to comply with the standards repeatedly? The biggest challenge remains the overt reliance on outdated systems and processes and manual labor. The others we have enumerated below.
- Lack of a gold standard for data. Sources of data have grown exponentially and the formats in which they are found have diversified over the years. Further, the widespread use of digital currency has increased the risks of money laundering phenomenally.
- Outdated and incomplete documentation: Data has grown prolifically. This makes customer profiling and integrating data from multiple sources more demanding than ever before. In the absence of automation, it becomes a time-consuming exercise. Most systems used for AML processing are extremely limited in scope and cannot scale as rapidly as desired or have clarity in terms of data accuracy.
- Gaps/flaws in AML-IT infrastructure and false positives: As evident in the instances of NatWest which risked censure for failing to make their systems robust, failure to raise timely alerts could be expensive. However, if the AML systems are not able to distinguish between illegal and legitimate transactions and notify good transactions as well, it loses its veracity. False positives result in duplication of effort and resultant wastage of time.
- External factors such as WFH and digitization: Rapid advance in digitization and WFH culture post the coronavirus pandemic has increased the threat landscape. Sophisticated means for money laundering like structuring and layering (adopted by criminals/frauds) require exceptional intelligence. However, many of the existing systems are not able to distinguish between illegal and legal transactions, let alone spot activities such as smurfing, where small cash deposits are made by different people.
- Human factors: When firms are dependent on manual labor, they will face certain typical problems. One of them is the late filing of suspicious activity reports (SAR). Then there is the case of bias and employee conflict of interest. We have the example of NatWest being fined £265 million where employee bias was evident in the laundering of nearly £400 million.
- Investment: Newer and tougher AML reforms call for investment in technology as existing systems are not sophisticated enough. But with the volatility in the market continuing unabated and worsening geopolitical crisis, that is tough.
Simplifying the Complexity of Compliance with Magic FinServ
Magic FinServ brings efficiency and scalability by automating AML operations using Magic DeepSightTM.
Magic DeepSightTM is an extremely powerful tool that on the one hand extracts data from relevant fields in far less time than before and on the other checks and monitors for discrepancies from a plethora of complex data existing in diverse formats and raises alerts in a timely manner. Saving you fines for late filing of suspicious activity report (SAR) and ensuring peace of mind.
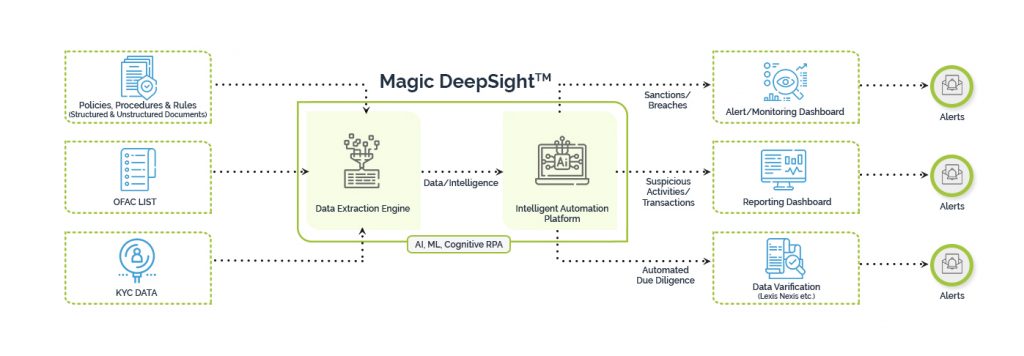
Customer due diligence: Before onboarding a new customer as well as before every significant transaction, banks and financial institutions must ascertain if they are at substantial risk for money laundering or dealing with a denied party.However, conductingchecks manually prolongs the onboarding and due diligence process, and is the leading cause of customer resentment and client abandonment.
We simplify the process and make it smoother and scalable. Our solution is powered by AI and RPA which makes the AML process more efficient in monitoring, detecting, reporting, and investigating money laundering and fraud along with compliance violations across the organization.
Magic DeepSightTM scours through the documents received from the customer during the onboarding process for onboarding and due diligence. These are verified against external sources for accuracy and for establishing credibility. Information is compared with credit bureaus to establish credit scores and third-party identity data providers to verify identity, Liens, etc.
Sanctions/Watchlist screening: One of the most exhaustive checks is the sanctions or the watchlist screening which is of paramount importance for AML compliance. The OFAC list is an extremely comprehensive list, that looks for potential matches on the Specially Designated Nationals (SDN) List and on its Non-SDN Consolidated Sanctions List.
Magic FinServ simplifies sanctions compliance. Our powerful data extraction machine and intelligent automation platform analyze tons of data for watchlist screening, with the least possible human intervention. What could take months is accomplished in shorter time spans and with greater accuracy and reduced operational costs.
Transactions monitoring: As underlined earlier, there are extremely sophisticated means for carrying out money laundering activities.
One of them is layering, where dirty money is sneaked into the system via multiple accounts, shell companies, etc. The Malaysian unit of Goldman Sachs was penalized with one of the biggest fines of 2020 for its involvement in the 1MBD scandal, where several celebrities were the beneficiaries of the largesse of the fund. This was the first time in its 151-year-old history that the behemoth Goldman Sachs had pleaded guilty to a financial violation. It was fined $ 600 million. The other is structuring where instead of a lump sum deposit (large), several smaller deposits are made from different accounts.
Magic DeepSightTM can read the transactions from the source and create a client profile and look for patterns satisfying the money laundering rules.
Reducing false positives: Magic DeepSightTM uses machine learning to get better in the game of distinguishing legal and illegal transactions in time. As a result, businesses can easily affix rules to lower the number of false positives which are disruptive for business.
KYC: KYC is a broader term that includes onboarding and due diligence and ensuring that customers are legitimate and are not on Politically Exposed Persons (PEPs) or sanctions lists. Whether it is bank statements, tax statements, national ID cards, custom ID cards, or other unique identifiers, Magic DeepSightTM facilitates a compliance-ready solution for banks and fintechs. You not only save money, but also ensure seamless transactions, reduce the incidences of fraud, and not worry about poor customer experience.
What do you gain when you partner with Magic FinServ?
- Peace of mind
- Streamlined processes
- Comprehensive fraud detection
- Minimum reliance on manual, less bias
- Cost efficiency and on-time delivery
- Timely filing of SAR
The time to act is now!
The costs of getting AML wrong are steep. The penalties for non-compliance with sanctions are in millions. While BitMex and NatWest have paid heavy fines – BitMex paid $ 100 million in fines, others like Credit Sussie suffered a serious setback in terms of reputation. Business licenses could be revoked. Firms also stand to lose legit customers when the gaps in their AML processes get exposed. No one wants to be associated with financial institutions where their money is not safe.
The astronomical AML fines levied by regulators indicate that businesses cannot afford to remain complacent anymore. AML fines will not slowdown in 2022 as the overall culture of compliance is poor and the existing machinery is not robust enough. However, you can buck the trend and avoid fines and loss of reputation by acting today. For more about our AML solutions download our brochure and write to us at mail@magicfinserv.com.