Home » Preparing your Asset Management firm to adopt AI for Back Office Operations
INSIGHTS
Intelligent solutions. Informed decisions. Unrivaled results.
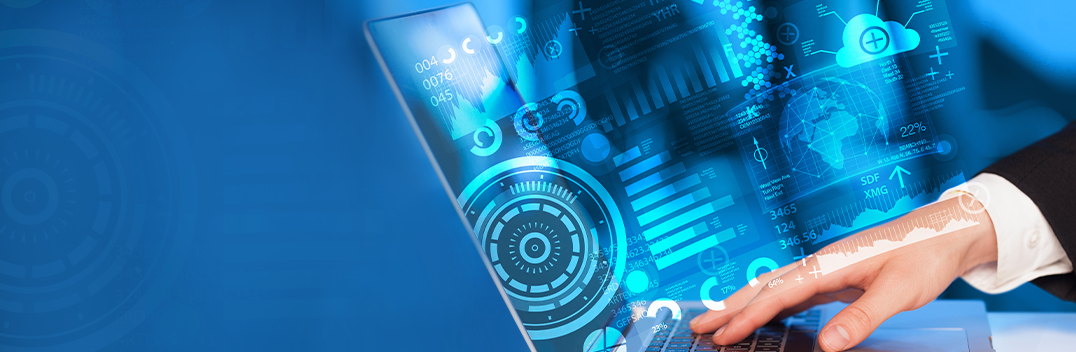
Preparing your Asset Management firm to adopt AI for Back Office Operations
Enterprises have increasingly realized that they must implement AI to succeed as digital natives are fast outpacing the ones relying on monolithic architectures. However, lack of synchronization between downstream and upstream elements, failure to percolate the AI value and culture in the organization’s internal dynamics, unrealistic business goals, and lack of vision often means that the AI projects either get stuck in a rut or fail to achieve the desired outcomes. What seemed like a sure winner in the beginning soon becomes an albatross around one's neck.
Mitigating the pitfalls with a well-drawn and comprehensive AI roadmap aligned to company needs
According to a Databricks report, only one in three AI and predictive analytics projects are successful across enterprises. Most AI projects are time-taking - it takes six months to go from the concept stage to the production stage. Most executives admit that the inconsistencies in AI adoption and implementation stems from inconsistent data sets, silos, and lack of coordination between IT and management and data engineers and data scientists. Then there’s the human element that had to be taken into account as well. Reluctance to invest, lack of foresight, failure to make cultural changes are as much responsible for falling short of the AI targets as the technical aspects enumerated earlier.
This blog will consider both the technical and the human elements vital for conducting a successful AI journey. To mitigate any disappointment that could accrue later, enterprises must assess the risk appetite, ensure early wins, get the data strategy in place, drive real-time strategic actions, implement a model and framework that resonates with the organization’s philosophy while keeping in mind the human angle - ensuring responsible AI by minimizing bias.
Calculating the risk appetite – how far the organization is willing to go?
Whether the aim is to enhance customer experience or increase productivity, organizations must be willing to do some soul searching and find out what they are seeking. What are the risks they are prepared to take? What is the future state of readiness/ AI maturity levels? And how optimistic are things at the ground level?
From the utilitarian perspective, investing in a completely new paradigm of skills and resources which might or might not result in ROI (immediately) is debatable. However, calamities of a global scale like COVID-19 demand an increased level of preparedness. Businesses that cannot scale up quickly can become obsolete; therefore, building core competencies with AI makes sense. Automating processes mitigates the challenges of the unforeseeable future when operations cannot be reliant on manual effort alone. So even if it takes time to reach fruition, and all projects do not translate into the desired dividends, it is a risk many organizations willingly undertake.
There is a lot at stake for the leadership as well. Once AI is implemented, and organizations start to rely on AI/ML increasingly, the risks compound. Any miscalculation or misstep in the initial stages of AI/ML adoption could cause grievous damage to the business’s reputation and its business prospects. Therefore, leadership must gauge AI/ML risks.
Importance of early wins – focussing on production rather than experimentation.
Early wins are essential. It elicits hope across an organization. Let us illustrate this with an example from the healthcare sector – the ‘moon shot’ project. Launched in 2013 at the MD Anderson Cancer Centre, the ‘moon shot project’ objective was to diagnose and recommend treatment plans for certain forms of cancer using IBM’s Watson cognitive system. But as the costs spiraled, the project was put on hold. By 2017, “moon shot” had accumulated costs amounting to $62 million without being tested on patients. Enough to put the management on tenterhooks. But around the same time, other less ambitious projects using cognitive intelligence were showing remarkable results. Used for simple day-to-day activities like determining if the patient needed help with bills payment and making reservations, AI drove marketing and customer experience while relieving back-officer care managers from the daily grind. MD Anderson has since remained committed to the use of AI.
Most often, it makes sense to start with process optimization cases. When a business achieves an efficiency of even one percent or avoids downtime, it saves dollars – not counting the costs of workforce and machinery. It is relatively easy to calculate where and how we can ensure cost savings in existing business cases instead of exploring opportunities where new revenue can be driven, as illustrated by the MD Anderson Cancer Centre case study. As we already know how the processes operate, where the drawbacks are, it is easier to determine areas where AI and ML can be baked for easy wins. The data is also in a state of preparedness and requires less effort.
In the end, the organization will have to show results. They cannot experiment willy-nilly. It is the business impact that they are after. Hence the “concept of productionize” takes center stage. While high-tech and glamorous projects look good, these are best bracketed as “aspirational.” Instead, the low-hanging fruit that enables easy gains should be targeted first.
The leadership has a huge responsibility, and to prioritize production, they must work in tandem with IT. Both should have the same identifiable business goals for business impact.
Ensuring that a sound data strategy is in place – data is where the opportunity lies!
If AI applications process data a gazillion times faster than humans, it is because of the trained data models. Else, AI apps are ordinary software running on conventional code. It is these amazing data models trained to carry out a range of complex activities and embedding NLP, computer vision, etc., that makes AI super-proficient. As a result, the application or system can decipher the relevant text, extract data from images, generate natural language, and carry out a whole gamut of activities seamlessly. So if AI is the works, data is the heart.
Optimizing data pool
Data is the quintessential nail in the absence of which all the effort devised for drafting an operating model for data and AI comes to naught. Data is the prime mover when it comes to devising an AI roadmap. For data to be an asset, it must be “findable, accessible, interoperable, and reusable”. If it exists in silos, data ceases to be an asset. It is also not helpful if it exists in different formats. It is then a source of dubiety and must be cleaned and formatted first. Without a unique identifier (UID), attached data can create confusion and overwrite. What the AI machinery needs is clean, formatted, and structured data that can easily be baked on existing systems. Data that can be built once and used in many use cases is fundamental to the concept of productized data assets.
It serves to undertake data due diligence or an exploratory data analysis (EDA). Find out where data exists, who is the owner, how it can be accessed, linkages to other data, how it can be retrieved, etc., before drawing out the roadmap.
The kind of data defines the kind of machine learning model that can be applied, for example, for supervised machine learning models, data and labels are essential for enabling the algorithm to draw an inference about the patterns in the label, whereas unsupervised learning comes when data does not have labels. And transfer learning when the data that an existing machine learning model has learned is used to build a new use case.
Once the data has been extracted, it must be validated and analyzed, optimized, and enriched by integrating it with external data sources such as those existing online or in social media and to be fed into the data pipeline. A kind of extract, transform and load. However, if it is done manually, it could take ages and still be biased and error-prone.
Drawing the data opportunity matrix to align business goals with data
Once the existing data has been sorted, find how it can be optimized for business by integrating it with data from external sources. For this purpose, an opportunity matrix, also known as the Ansoff matrix comes in handy. A two-by-two matrix that references new business and current business with the data subsets (internal and external), it aids the strategic planning process and helps executives, business leaders understand where they are in terms of data and how they would like to proceed further.
Driving real-time strategic actions for maximum business impact using AI: Leadership matters
Real-time strategic actions are important. For example, millennial banks and financial institutions must keep pace with customer expectations or else face consequences. By making the KYC process less painstaking with AI, banks and FinTechs can drive unexpected dividends. When the KYC is done manually, it is time taking. By the time the KYC is complete, the customer is frustrated. When AI and Machine Learning capabilities are applied to existing processes, organizations reduce manual effort and errors substantially. The costs of conducting the KYC are reduced as well. However, the biggest dividend or gain that organizations obtain is in the customer experience that rebounds once the timelines ( and human interaction) are reduced. That is like having the cake and eating it too!
SAAS, on-prem, open-source code - finding out what is best!
If it is the efficiency and customer experience that an enterprise is after, SaaS works best. Hosted and maintained by a third party, it frees the business from hassles. However, if one wants complete control over data and must adhere to multiple compliance requirements, it is not a great idea. On-prem, on the other hand, offers more transparency and is suitable for back-end operations in a fintech company for fast-tracking processes such as reconciliations and AML/KYC. Though SaaS is feasible for organizations looking for quality and ease of application, open-source code produces better software. It also gives control and makes the organization feel empowered.
Conclusion: AI is not a simple plug and play
AI is not a simple plug-and-play. It is a paradigm shift and not everyone gets it right the first time. Multiple iterations are involved as models do not always give the desired returns. There are challenges like the diminishing value of data which would require organizations to broaden their scope and consider a wider data subset for maximizing accuracy.
Notwithstanding the challenges, AI is a proven game-changer. From simplifying back-office operations to adding value to day-to-day activities, there is a lot that AI can deliver. Expectations, however, would have to be set beforehand. The transition from near-term value to closing in on long-term strategic goals would require foresight and a comprehensive AI roadmap. For more information on how your organization could use AI to drive a successful business strategy, write to us at mail@magicfinserv.com to arrange a conversation with our AI Experts.