Gartner Says By 2020, a Corporate “No-Cloud” Policy Will Be as Rare as a “No-Internet” Policy Is Today.
With the increasing number of cloud adoption rate, it has become instrumental for organizations to build a robust Cloud migrations strategy.
As per the Commvault report. the cloud Fear of Missing Out (FOMO) is driving the business leaders to move full speed ahead towards the cloud.
Many organizations are already moving part of their applications to cloud or planning to move all of their applications to cloud. Apart from the reliability, scalability, cost optimization and security benefits, the recent disruption in cognitive technologies like AI/ML/Blockchain are one of the driving factors to embrace the cloud as an important IT strategy Most of the cloud providers are offering attractive easy to implement AI/ML platform along with other multidimensional benefits.
However, there are so many cloud providers, so many cloud services are available in the market.
Who is the best service provider? Which service model should be fit for the organizations?
The answers are not a single word or list of words. This is a process appropriately designed towards your business goals.
Hence choose a Cloud service provider who can work as a partner, not as a vendor.
This is a journey through the learning curve for both partners.
I am highlighting a few aspects which must be considered when selecting a cloud partner:
Define your migration strategy – IaaS vs PaaS vs SaaS. You need to select the right partner for platform, infrastructure and application services. Sometimes you may need to work with multiple providers for different services or you can have one combine managed Service partner.
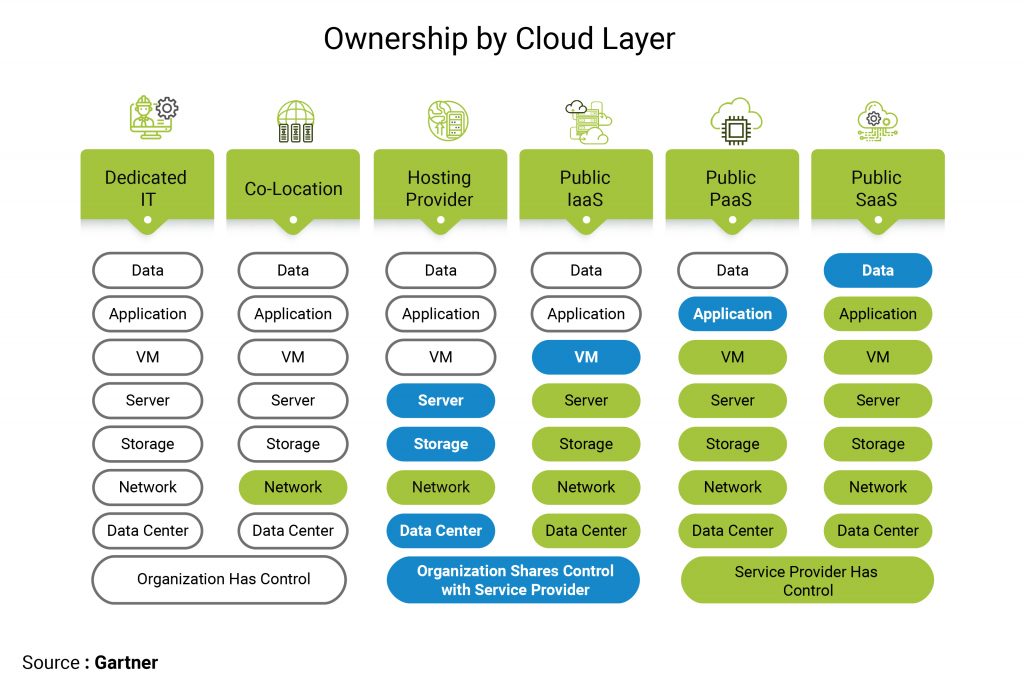
The above diagram from Gartner is showing a perfect ownership sharing in various cloud service model. Like if you have a best in class application service team, you can procure infrastructure services or platform services from a service provider and align the internal team to run the cloud service. This will need extensive cloud training for the existing team, hire some cloud experts to build the in-house capability and robust service management process to coordinate among different vendors. Your cloud partner should take a role of your training partner in such cases. In a SaaS-based model, this is essential that cloud partner know your business and industry well. Because ultimately the cloud service will be fully integrated with the business model. Hence it is very important that the SaaS provider is fully aligned with your business need. Overall Selection Criteria can be designed by analyzing and comparing the below factors-
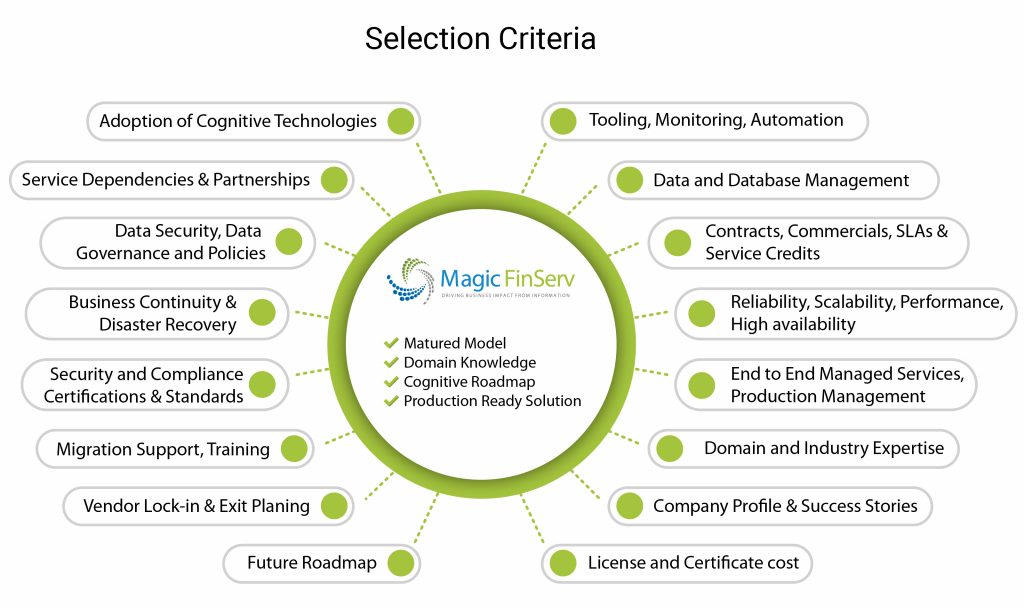
The provider must be knowledgeable about your application, data, interfaces, compliance, security, BCP/DR and other business requirements. Critical Success Factors are defined in the various model. However, as per our study, we have listed Seven key success factors for cloud computing –
Cloud Partner – The most important step towards success. Choose a perfect cloud partner who will help in your journey towards success
Cloud Strategy –
- Create a plan & solution architecture
- Define the cloud applications and services
- Prepare the service catalogue
- Build the capability and processes
Cost & performance – one of the most important success criteria.
- Plan cost and ROI
- Benchmark the performance
- Proactive monitoring
- Capacity planning
- Right-sizing & optimization
Security –
- Build the security strategy – secure all the layers and components
- Automation, tooling and proactive monitoring
- Plan the audit, compliance reporting & certification
Contract & SLA –
- Incorporate all the aspects of the contract carefully with the legal help
- Build customer and suppliers terms properly
- Define Service SLA & service credits
- Manage the contract (an ongoing process)
Automation –
- Have an automation strategy
- From Infrastructure to Application – automate the repetitive work
- Increase the response and resolution
- Reduce the human error
Manage the stakeholders –
- Cloud adoption changing the organizational structure and IT landscape drastically.
- Manage your stakeholders throughout the journey.
- Assess the impact of positive and negative stakeholders on the project.
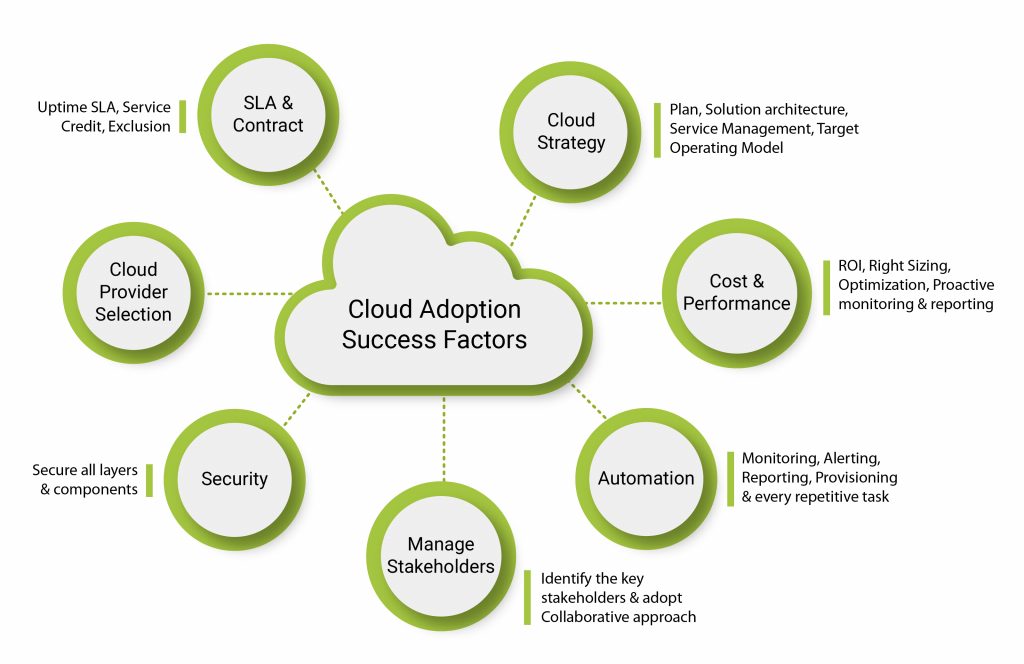
A managed service provider is the ideal solution in today’s complex world. At MagicFinServ, we are helping the global FinTech companies to build their successful SaaS model. Our highly skilled cloud team can align all the moving parts from architecting to implementation and deliver a production-ready solution. To know more about our Financial services focused cloud solution please contact us at mail@magicfinserv.com.