Home » Data Consolidation Simplified with DCAM & Data Control Environment
INSIGHTS
Intelligent solutions. Informed decisions. Unrivaled results.
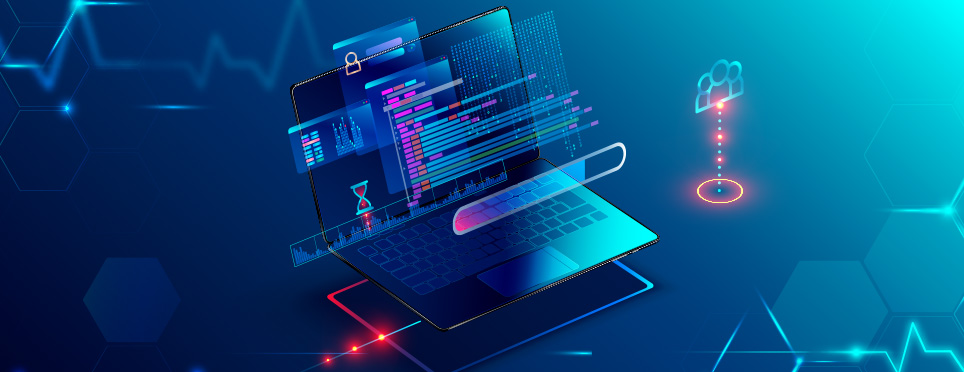
Data Consolidation Simplified with DCAM & Data Control Environment
In the good old days, an organization’s ability to close its books in time at the end of the financial year was a test of its data maturity. The mere presence of a standard accounting platform was not sufficient to close books in time. As CFOs struggled to reduce the time to close from months to weeks and finally days, they realized the importance of clean, consolidated data that was managed and handled by a robust Data Execution framework. This lengthy, tiresome and complex task was essentially an exercise of data consolidation - the "closing of the records" or setting the records straight. Data as per the Oxford Dictionary of Accounting is quite simply a "procedure for confirming the reliability of a company’s accounting records by regularly comparing (balances of transactions).”
From the business and financial perspective, the closing of records was critical for understanding how the company was faring in real-time. Therefore, data had to be accurate and consolidated. While CFOs were busy claiming victory, the Financial Institutions continued to struggle with areas such as Client Reporting, Fund Accounting, Reg Reporting and the latest frontier, ESG Reporting. This is another reason why organizations must be extremely careful while carrying out data consolidation. The regulators are not just looking more closely into your records. They are increasingly turning vigilant and digging into the details and questioning omissions and errors. And most importantly, they are asking for an ability to access and extract data themselves, rather than wait for lengthy reports.
However, if there are multiple repositories where you have stored data, with no easy way to figure out what that data means - no standardization and no means to improve the workflows where the transactions are recorded, and no established risk policy – how will you effectively manage data consolidation (a daily, monthly, or annual exercise) let alone ensure transparency and visibility.
In this blog, we will argue the importance of data governance and data control environment for facilitating the data consolidation process.
Data governance and the DCAM framework
By 2025, 80% of data and analytics governance initiatives focused on business outcomes, rather than data standards, will be considered essential business capabilities.
Through 2025, 80% of organizations seeking to scale digital business will fail because they do not take a modern approach to data and analytics governance. (Source: Gartner)
In some of our earlier blogs, we have emphasized the importance of data governance, data quality, and data management for overall organizational efficiency. Though these terms sound similar, they are not quite the same.
As per the DCAM framework – a reliable tool for assessment and benchmarking of an organization's data management capabilities, Data Management, Data Quality, and Data Governance are distinctly separate components. While Data Management Program and Funding forms the core – the foundation; Data Quality Management and Data Governance are the execution components with Data Control Environment as a common thread running between the other core execution elements. (See: DCAM framework)
For high levels of data maturity, something that is highly sought by financial institutions and banks, democratization and harmonization or consolidation of the data elements are necessary. This quite simply means that there must be one single data element that is appropriately categorized/classified and tagged, instead of the same existing in several different silos. Currently, the state of data in a majority of banks and financial institutions is such that it inspires little trust from key stakeholders and leading executives. When surveyed, not many asserted confidences in the state of their organization's data.
For ensuring high levels of trust and reliability, robust data governance practices must be observed.
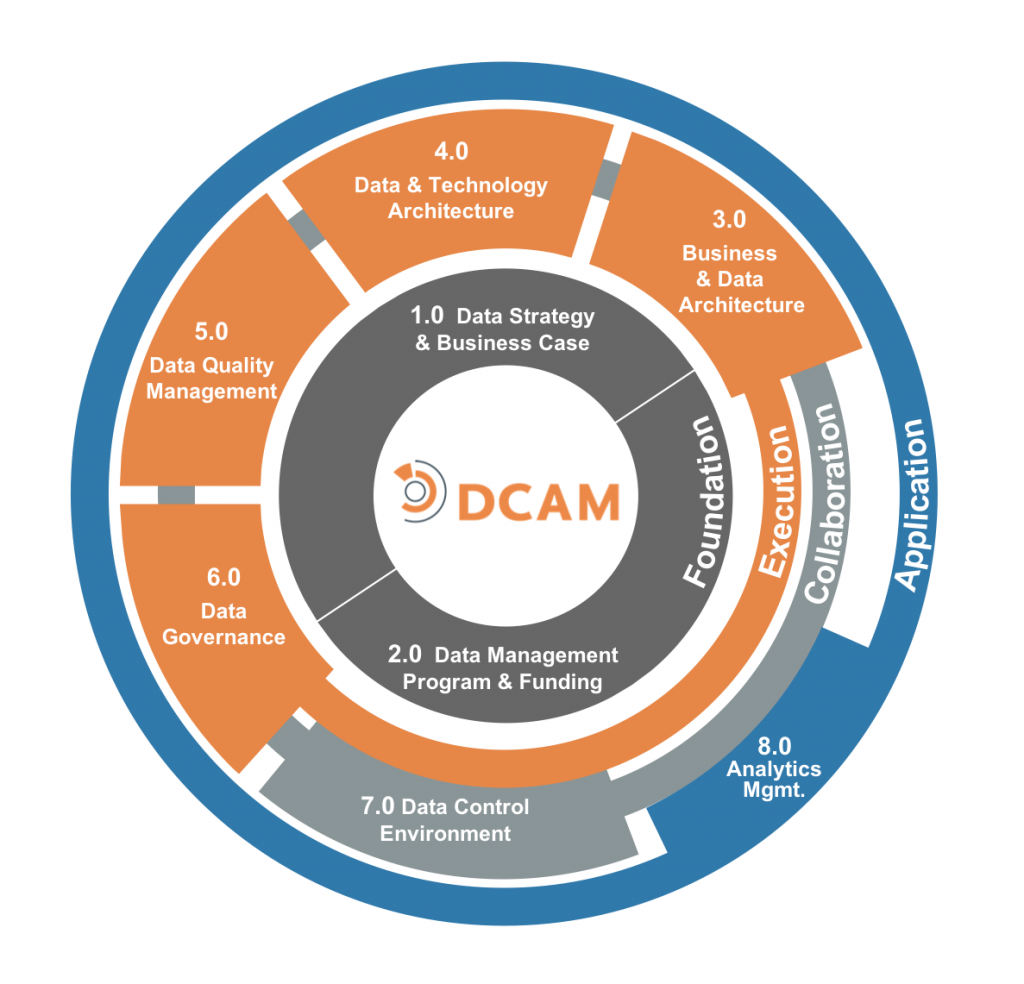
Getting started with Data Control
Decoding data governance, data quality, and data control
So, let's begin with the basics and by decoding the three…
Data Governance: According to the DCAM framework - the Data Governance (DG) component is a set of capabilities to codify the structure, lines of authority, roles & responsibilities, escalation protocol, policy & standards, compliance, and routines to execute processes across the data control environment.
Data Quality: Data Quality refers to the fitment of data for its intended purpose. When it comes to Data Quality and Data Governance, there's always the question of what came first – data quality or data governance. We'll go with data governance. But before that, we would need a controlled environment.
A robust data control environment is critical for measuring up to the defined standards of data governance, and for ensuring trust and confidence amongst all the stakeholders involved that the data they are using for fueling their business processes and for decision making is of the highest quality. Also, there is no duplication of data, the data is complete, error-free and verified, and accessible to the appropriate stakeholder.
For a robust data control environment:
- Organizations must ensure that there is no ambiguity when it comes to defining key data elements.
- Data is precisely defined. It must have a meaning - described with metadata (business, operations, descriptive, administrative, technical) to ensure that there is no ambiguity organization-wide.
- Secondly, data, whether it is of clients, legal entities, transactions, etc., must be real in the strictest sense of the term. It must also be complete – definable, for example AAA does not represent a name.
- Lastly, data must be well-managed across the lifecycle as changes/upgrades are incorporated. This is necessary as consolidation is a daily, monthly, or annual exercise and hence the incorporation of the changes or improvements in the workflows is necessary for real-time updates.
But what if a data control environment is lacking? Here are the multiple challenges that the organization will face during data consolidation:
- As there are multiple departments with their own systems, there are multiple spreadsheets as well.
- Due to the inconsistencies and inability to update workflows - operational and financial data might differ.
- Mapping and cross-referencing of data will be tedious as the data exists in silos.
- If there are inaccuracies that must be sorted, they will be reflected in standalone worksheets…no single source of truth will prevail.
- Quite likely that ambiguities will still exist even after the consilidation exercise is over.
- Meeting compliance and regulatory requirements would require expending manpower again as there is little to no transparency and visibility.
- The focus will not be as much on the process as on ensuring high levels of data quality and elimination of waste.
- Data nomenclature: data defined against predefined requirements, so it is easier to extract relevant data.
- With automation and standardization, data owners and consumers get the benefit of targeted information - Variances are recorded and made available to the right people.
- Information is shared/accessible to everyone who needs to know. Does not exist in silos anymore.
- Auditing becomes easy as there is visibility and transparency.
- With consolidation expediated, speedier decision-making ensues
Now compare this with what happens when you rely on robust governance and data control environment practices.
In short, with a robust data control environment and data governance practices, banks and FIs, can minimize consolidation efforts, time, and manpower, resulting in enhanced business opportunities and a greater degree of trust in the data amongst stakeholders.
Staying in control
Magic FinServ is a DCAM EDMC partner, its areas of specialization being the ability to manage offline and online data sources, the understanding of the business rules in financial services organizations and the ability to leverage APIs RPAs, allowing data to be moved across siloed application and business units, overcoming other gaps that could have led to data issues. Magic FinServ can bring in some of these techniques and ensure data control and data governance.
The DCAM framework is both an assessment tool and an industry benchmark. Whether it is the identification of gaps in data management practices or ensuring data readiness for minimizing data consolidation efforts, as an EDMC’s DCAM Authorized Partner (DAP) for providing a standardized process of analyzing and assessing your Data Architecture and overall Data Management Program, we'll aid you in getting control of data with a prioritized roadmap in alignment with the DCAM framework.
Further, when it comes to data - automation cannot be far behind. For smooth and consistent data consolidation that generates greater control over your processes while ensuring the reliability of the numbers, you can depend on Magic FinServ's DeepSightTM . For more information on the same contact us today at mail@magicfinserv.com